Introduction
The rapid advancement of artificial intelligence (AI) technology has permeated various aspects of modern society, including the field of education. As AI systems become increasingly sophisticated, their potential applications in the classroom setting have garnered significant attention from educators, researchers, and policymakers alike. Large Language Models, or LLMs, like ChatGPT or Claude, are types of AI that have the ability to interact with users in a responsive capacity for a variety of applications across almost any subject, including teaching and learning. One of the critical areas of interest is the usage of AI by students for educational purposes, which presents both opportunities and challenges.
The integration of AI in education has the potential to revolutionize the learning experience for students. AI-powered tools and platforms can provide personalized learning experiences tailored to individual students’ needs, learning styles, and abilities (Zawacki-Richter et al., 2019). For example, intelligent tutoring systems, which have been around for much longer than the new LLMs, can adapt to a student’s level of understanding and provide targeted feedback and support, fostering a more effective and engaging learning process (Beck, Stern, & Haugsjaa, 1996; Kulik & Fletcher, 2016).
A very recent study by the Walton Family Foundation (2024) that focused on parents, teachers, and students found that in the past year, AI has become deeply integrated into the education system, with increasing awareness by teachers, parents, and students and a roughly 50% usage rate across all three groups. Despite a rise in negative perceptions, the general sentiment among these groups remains positive, particularly among those who have personally used AI and see its beneficial applications in educational settings.
According to a Rand study (2024), as of fall 2023, 18 percent of K–12 teachers regularly used AI for teaching purposes, and another 15 percent had experimented with AI at least once. AI usage was more prevalent among middle and high school teachers, especially those teaching English language arts or social studies. Most teachers who integrated AI into their teaching employed virtual learning platforms, adaptive learning systems, and chatbots on a weekly basis. The primary applications of these AI tools included customizing instructional content to meet students’ needs and generating educational materials.
By the conclusion of the 2023–2024 academic year, 60 percent of school districts aim to have provided AI training for teachers, with urban districts being the least likely to implement such training. In interviews, educational leaders expressed a stronger emphasis on encouraging AI adoption among teachers rather than formulating policies for student use, citing the potential of AI to streamline teachers’ tasks.
Most other current research on the effectiveness of AI in education is focused on adult learners and generally leans towards the positive when concerned with achievement and perceptions (Kumar & Raman, 2022; Zheng et al., 2023).These studies also show that adult students believe AI is best served for usage by students and teachers in assistance of assignment creation or completion, as well as tutoring systems or research assistants as opposed to automated assessment use cases.
However, most K-12 teachers, parents, and students feel that their schools are not adequately addressing AI. They report a lack of policies, insufficient teacher training, and unmet demands for preparing students for AI-related careers. This absence of formal school policies means that AI is often used without official authorization, leaving students, parents, and teachers to navigate its use independently. There is a strong preference among all stakeholders for policies that explicitly support and thoughtfully integrate AI into education.
Emerging literature indicates that students have varying opinions on the usage of AI tools, and that AI has the potential to assist students in learning and ultimately increase achievement, regardless of whether the learners are adults or children (Martínez, Batanero, Cerero, & León, 2023; Trisoni et. al, 2023).
Furthermore, AI can assist students in various tasks, such as information retrieval, research, writing, and problem-solving. Language models like ChatGPT, developed by OpenAI, have demonstrated remarkable capabilities in generating human-like text, potentially aiding students in their writing assignments and research projects (Bender et al., 2021; Zheng, Niu, Zhong, & Gyasi, 2023). Additionally, AI-powered virtual assistants can provide on-demand support and guidance, acting as digital tutors or study companions (Winkler & Söllner, 2018; Chen, Jensen, Albert, Gupta, & Lee, 2023).
However, AI use by students also raises ethical concerns and potential risks. One of the primary concerns is the potential for academic dishonesty, as students may be tempted to use AI-generated content as their own, raising issues of plagiarism and cheating (Driessen & Gallant, 2022; McGehee, 2023;). There is also a risk of AI systems perpetuating biases and misinformation, as these systems are trained on existing data that may reflect societal biases or contain inaccuracies (Mehrabi et al., 2021).
Moreover, the increasing reliance on AI tools may impact students’ critical thinking and problem-solving skills, as they become more dependent on these systems for tasks that traditionally required human reasoning and creativity (Luckin et al., 2016). Additionally, there are concerns about the potential for AI to widen the digital divide, as access to advanced AI tools and resources may be limited for certain socioeconomic groups, potentially exacerbating existing educational inequalities (Selwyn, 2019).
Significance of the Study
This study holds significant importance for several reasons. It is the second study by Michigan Virtual in the field of artificial intelligence and education. It seeks to be a companion piece to work done with educators and help inform current and future work in the field as a thought leader and the space of artificial intelligence and education.
Firstly, it addresses a rapidly evolving technological landscape that is reshaping education. As AI advances and becomes more integrated into various aspects of society, it is crucial to understand its implications for the education sector, particularly from the perspective of students, who are the primary consumers of educational services.
Second, this study intends to fill a gap in the literature by examining how students utilize AI (use cases), what they think about AI (perceptions), their frequency of AI usage, and how these individual or grouped variables relate to demographic characteristics and the almighty student outcome of achievement or grades.
Third, this study’s findings have the potential to inform policymakers, educational institutions, and educators about the current state of AI adoption among students. By understanding students’ motivations, perceptions, and experiences with AI tools, stakeholders can develop strategies and policies that promote responsible and effective AI usage in educational settings. This knowledge can guide the development of guidelines, best practices, and training programs to ensure that AI is leveraged in a manner that enhances learning outcomes while mitigating potential risks and ethical concerns.
Finally, the insights gained from this study can contribute to the broader discourse on the role of AI in education and its implications for pedagogical approaches, curriculum design, and the future of teaching and learning. As AI becomes more integrated into educational processes, it is crucial to critically examine its impact on traditional teaching methods, assessment strategies, and the overall learning experience. The study’s findings can inform discussions and decision-making processes related to the effective integration of AI in a manner that complements and enhances human-centric educational practices.
In summary, this study’s significance lies in its potential to inform policies, practices, and decision-making processes related to the responsible and equitable integration of AI in education. Current research indicates that AI tools may have multiple benefits, including positive impacts on achievement.
Research Questions
This study used several research questions to fulfill the purpose of understanding online student usage habits of AI, opinions regarding AI, and any relationships those key factors may have with outcome and demographic variables such as achievement, grade level, or course subject area.
- What are students’ AI Usage Habits?
- Are there differences in student achievement based on AI usage?
- Are there differences in student perceptions of AI?
- What differences exist in student usage of AI?
Methodology
To address the research questions and fulfill the purpose of this study, a causal-comparative approach was used, meaning that no treatment was applied, no groups were randomized, and all of the data utilized already existed within Michigan Virtual databases.
Data utilized were collected over a period of 1-2 months in early 2024 and consisted of LMS data and End of Course survey data.
Variables and Instrumentation
The data for this study were composed of variables created from two different instruments and are described in detail below. Each data source was anonymized and matched by using unique student ID codes.
LMS and Publicly Available Data
Data from the LMS and publicly available district information was matched with End of Course Survey data, and included the following information on students:
- Course Grades
- Number of Current Enrollments at Michigan Virtual
- IEP Status
- Course Type (Remedial, AP, etc)
- Course Subject
- Public District Information – district size, free and reduced lunch proportions
- Locale – defined by population density in four categories
- SES – percentage of students in a district that qualify for free or reduced lunch, divided into four categories. This is not an individual student’s socioeconomic status, but rather a summary of the district’s status that the student belongs to.
- The thresholds for the categories are as follows:
- Low – Below 25%
- Moderate – Between 25 and 50%
- High – 50% to 74%
- Very High – 75% and above
- The thresholds for the categories are as follows:
All of these data points were used as variables in this study. The number of Current Enrollments, IEP Status, and Course Type are all variables that historically have been used as covariates and moderating variables when predicting student achievement with Michigan Virtual students, and thus were also used in this study when achievement was the dependent variable in question.
End of Course Survey
Data from student End of Course surveys were matched with LMS data, and included the following information on students:
- Satisfaction
- Ease of Use (Predictor Variables)
- Comprised of multiple items regarding the ease of use of the learning platform
- Teacher Responsiveness and Care (Predictor Variables)
- Comprised of multiple items regarding teacher/student communication
- Prior Experience and Effort (Predictor Variables)
- Comprised of multiple items that summarize a student’s prior experience with online learning and general effort put towards learning.
- Tech Issues (Predictor Variables)
- Comprised of multiple items that indicate any difficulties or problems students may have had in the course from a technical standpoint, and if/how they were resolved.
- AI Usage, AI Use Cases, and AI Perceptions
Predictor Variables in this study, refer to variables that are included in certain analyses for Michigan Virtual because they have been previously identified as important in predicting student satisfaction and success.
While all of these variables were included in the exploratory phase of this study, not all information from the End of Course survey was included in the final analysis due to relevance or usefulness in model construction. The most important items extracted from the survey were the key variables of AI usage, AI Use Cases, and AI Perceptions. These are described in detail below.
- AI Usage
- This variable was captured by asking all students whether they have utilized AI during the completion of their online Michigan Virtual courses. Answers were binary Yes/No.
- Students were clearly told that this would be kept confidential and that it would in no way penalize them or affect their grades.
- AI Use Cases
- This variable set consisted of multiple select use cases for AI tools, and was only answered by students that answered “Yes” to the AI Usage item.
- Choices included:
- Summarizing information
- Conducting research/finding information
- Writing and editing assistant
- Explaining complicated concepts or principles in simpler terms
- Tutor/Teacher
- Create study guides or sample test questions
- Other (write-in)
- During the analysis, it became clear that there were two distinct groups that each of the choices could be categorized into: use as a tool and use as a facilitator. These two categories would be created and used in subsequent analyses, and is discussed at length later in the study, but also described below.
- Use as a facilitator – included selections that enabled students to still take on the main task of learning, rather than complete a very specific task itself.
- Use as a tool – included selections where students used AI for a very specific task to get a specific result: calculation, information retrieval, editing, summarization, etc.
- AI Perceptions
- This variable was captured by asking all students to share how they perceive the usefulness of AI tools in learning. This was recorded on a 5-point Likert-type scale ranging from Not Useful at All (1) to Extremely Useful (5), with a neutral (3).
Analysis Methods
This was a largely exploratory study addressing a multitude of research questions, each of which used a variety of data analysis techniques. To simplify the explanation, a table was created to match analytical methods and variables to research questions.
Table 1. Analysis Methods
Research Question | Analytical Methods | Key Variables |
---|---|---|
What are students’ AI Usage Habits? | Descriptive Statistics | AI Usage AI Perceptions AI Use Cases |
Are there differences in student achievement based on AI usage? | ANCOVAs Pearson R Correlations Linear Regression | Achievement AI Usage (1) AI Use Cases (2) Demographics |
Are there differences in student perceptions on AI? | ANCOVAs Pearson R Correlations | AI Perceptions AI Usage (1) AI Use Cases (2) Demographics |
What differences exist between student usage of AI? | ANCOVAs Pearson R Correlations | AI Usage AI Perceptions AI Use Cases Demographics |
Participants
This study included 2,154 students enrolled in Michigan Virtual courses in the fall of 2023 who completed an End of Course survey in early 2024.
Of the sample, approximately 50% of students were enrolled in a school district categorized as low proportions of free and reduced lunch students, and roughly 25% in moderate or high proportions of students with free and reduced lunch, and a slight presence of 1% of students enrolled in a district with very high amounts of students in free and reduced lunch programs
Most students also lived in suburban areas (50%), or rural town areas (35%), and around 10% resided in urban city districts.
Figure 1 below shows the distribution of students across their locale types and the approximate percentage of impoverished students in their district.
Figure 1. Student Distribution by Locale and Low SES Percentage
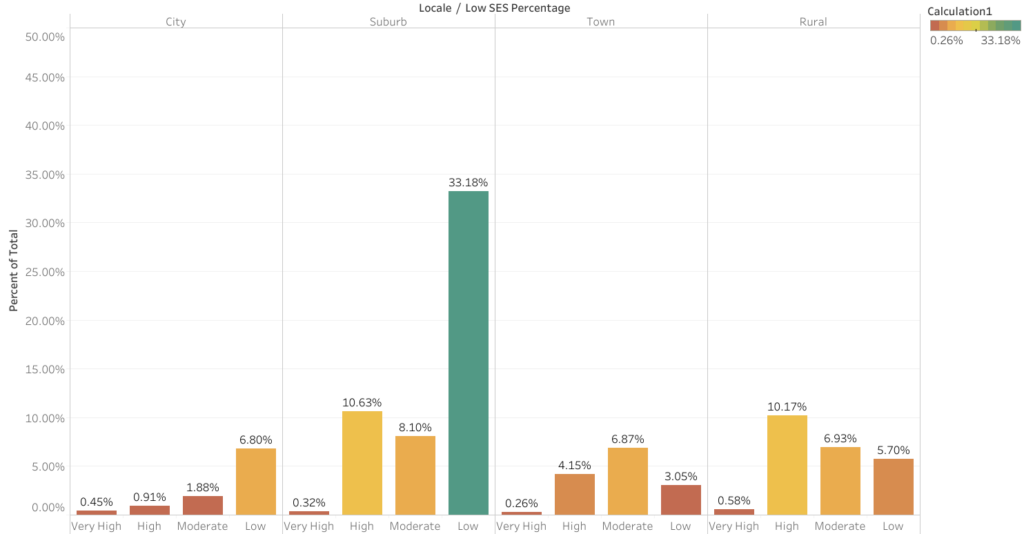
Over half of the students in the sample were in 11th (28%) or 12th (44.5%) grade, while 9th (7%) and 10th (17%) graders composed about a quarter of the sample. Middle school grades comprised about 4% of the students collectively, and elementary school students accounted for only about .5% of the students in the sample (one student). The supermajority of students were in high school, most heavily weighted towards the higher grade levels.
Outside of classes in the Other category, most of the students in the sample were enrolled in World Languages courses (22.5%), with Social Studies (14.7%), Science (12.4%), and Math (8.0%), comprising over a third of the student enrollments. A detailed breakdown can be seen below in Figure 2.
Figure 2. Subject Area Distribution
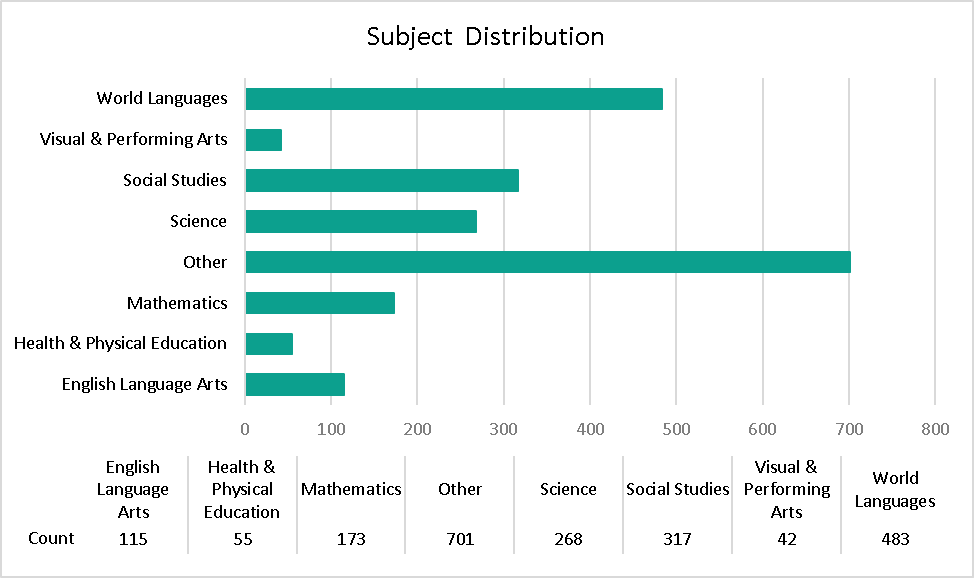
Furthermore, a cross-tabulation visual in Figure 3 of student grade level and subject shows the distribution of students’ grade levels across subjects.
Figure 3. Student Subject Area Distribution by Grade Level
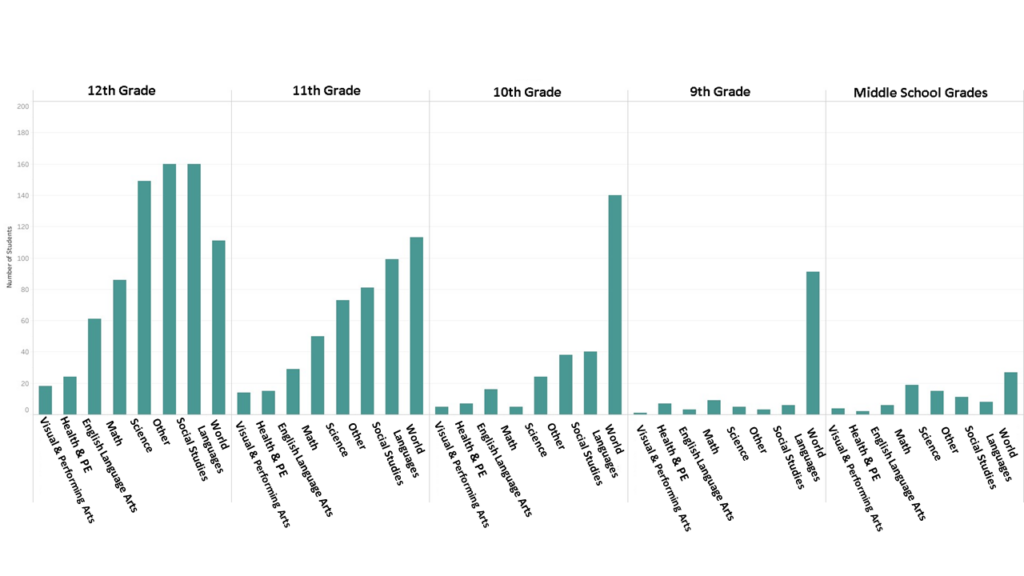
Regardless of grade, most students tended to be represented in the World Languages courses, with 12th and 11th graders also displaying comparable enrollments in Social Studies, Science, and Other courses.
Results
The results of the several different analyses will be discussed individually by the research question below and then synthesized in the next section. This section is limited to a presentation and interpretation of data.
Research Question 1: What are students’ AI Usage Habits?
This research question utilized descriptive statistics and cross-tabulations to analyze data from end-of-course survey items that addressed the following:
- AI usage – Yes/No response
- AI tools used – multiple select response of AI tools
- AI use cases – multiple response choices on reasons why students use AI
- AI perceptions – 5 point likert scale response on favorability of AI tools
These items were cross tabulated with demographic data to uncover any meaningful trends.
Results indicated that only around 8% (n = 166) of the 2,164 students reported using Artificial Intelligence tools for their courses, and ChatGPT was the most frequently used tool (77%).
Use cases for the AI tools amongst the students varied much more. As seen below in Table 2, the most frequent use of AI tools was, “To explain complicated concepts or principles in simpler terms,” followed closely by, “To conduct research or find information.”
The original 7 categories were then binned into the classifications of “facilitator” and “tool” for easier understanding and grouping.
Table 2. AI Use Case Bins
Use Case | N | Category | Percent of Total (%) |
---|---|---|---|
To explain complicated concepts or principles in simpler terms | 92 | Facilitator | 24.90% |
To conduct research/find information | 77 | Tool | 20.90% |
To summarize information | 65 | Tool | 17.60% |
To create study guides or sample test questions | 46 | Facilitator | 12.50% |
As a writing and editing assistant | 38 | Tool | 10.30% |
As tutor / teacher | 35 | Facilitator | 9.50% |
Other | 16 | —- | 4.30% |
Approximately 47% of students used AI tools as a “facilitator” and 48% used AI as a “tool”. It is important to note that no students reported using AI as a facilitator only, but rather always in conjunction with AI as “tool” usage.
This binning was used to help categorize students in subsequent analyses in order to better compare use cases of AI on several dependent variables.
Research Question 2: Are there differences in student achievement based on AI usage?
This research question utilized LMS data and end of course survey data in an ANCOVA model to address the research question in two ways: one model that addressed actual usage of AI tools, and then another that addressed use cases of those that did utilize AI.
Traditionally, achievement at Michigan Virtual is influenced by a student’s course load and IEP status, therefore these were used as covariates in the model. During the analyses, it was found that districts with disproportionately higher rates of free and reduced lunches performed worse in terms of students’ grades. This led to its inclusion as a covariate, with all analyses re-run to include it, in order to extract that variance. Its relationship with dependent variables is discussed further in the findings sections of this study.
The average grade percentage of all students in the dataset was 82.23, which was comparable to the population average established in Michigan Virtual’s yearly effectiveness report (Freidhoff, DeBruler, Cuccolo, & Green, 2024).
Model 1 – General Model
The general ANCOVA model (R2 =.470) included the scale measure dependent variable of student achievement, covarying on student IEP, number of courses, and product lines. Independent variables in the model included AI usage (yes/no), AI Opinions, Subject, Grade Level, SES, and Locale (population density).
This resulted in indicating a main effect of SES (p <.05, F = 2.939), with all other variables producing no main effects or multiple interaction effects. This indicated that, as discussed before, students who were enrolled in districts with higher percentages of free and reduced lunch students tended to achieve lower than their counterparts. This can be seen below in Table 3.
Table 3. Average Grades by Low SES Percentage
Low SES Percentage | Mean Grade | Std. Error |
---|---|---|
Low | 82.494 | 1.484 |
Moderate | 80.986 | 1.792 |
High | 80.429 | 1.703 |
Very High | 58.606 | 5.701 |
Students that resided in low percentages of free and reduced lunch students scored higher, and scores decreased as percentages of students with free and reduced lunch increased, with students in areas of Very High amounts of students with free and reduced lunch score much lower than the other groups of students.
This led to the revision of the model to include SES as covariate, as discussed above (R2 =.523). The model was then revised and resulted in an ANCOVA model that produced no main effect interactions, meaning that once SES was accounted for, no variables in the model had any significant impact on students’ grades.
Students who reported using AI (82.153) and students who reported not using AI (82.373) had almost identical grades. In addition, correlations between use of AI and regression models including the use of AI as a predictor alongside Michigan Virtual’s previously curated predictor variables, AI Use was found to have no significant relationship with achievement, and was not a significant predictor of achievement.
While no single variable had an impact on student grades, within-group observations indicated some significant variation of grades (p <.05) within the variable of AI Perceptions. For all students, regardless of whether they stated using AI or not, those students who held less extreme viewpoints on AI (2,3,4 on a five-point scale) scored higher than those who held highly unfavorable and highly favorable viewpoints (1 or 5).This can be seen in Table 3 and Figure 4 below.
Table 3. AI Perceptions and Average Grades
AI Perception | Mean Grade | Std. Error |
---|---|---|
Very Unfavorable | 83.289 | 3.065 |
Unfavorable | 86.328 | 2.847 |
Neutral | 84.516 | 1.881 |
Favorable | 81.536 | 2.220 |
Very Favorable | 76.107 | 2.607 |
This suggested that there may be a nonlinear relationship between the variables. Curve estimation results indicated the possibility of a quadratic relationship (parabolic shape), and while the relationship was significant (p<.05), the correlation was weak (.117).
Figure 4. Average Grades by AI Perception
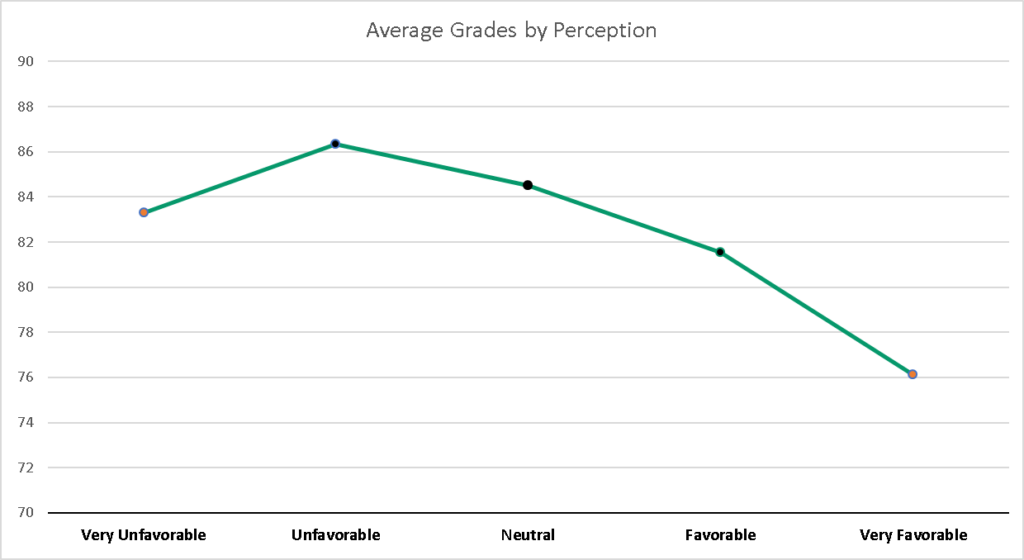
This type of relationship and curve was also present when including AI Usage, as both students who used AI and did not use AI achieved similar grades across their perception categories, which indicates that student perceptions of AI and its relationship with achievement is not due to whether a student uses AI or not. This can be seen below in Figure 5.
Figure 5. Average Grades by AI Perceptions and Usage
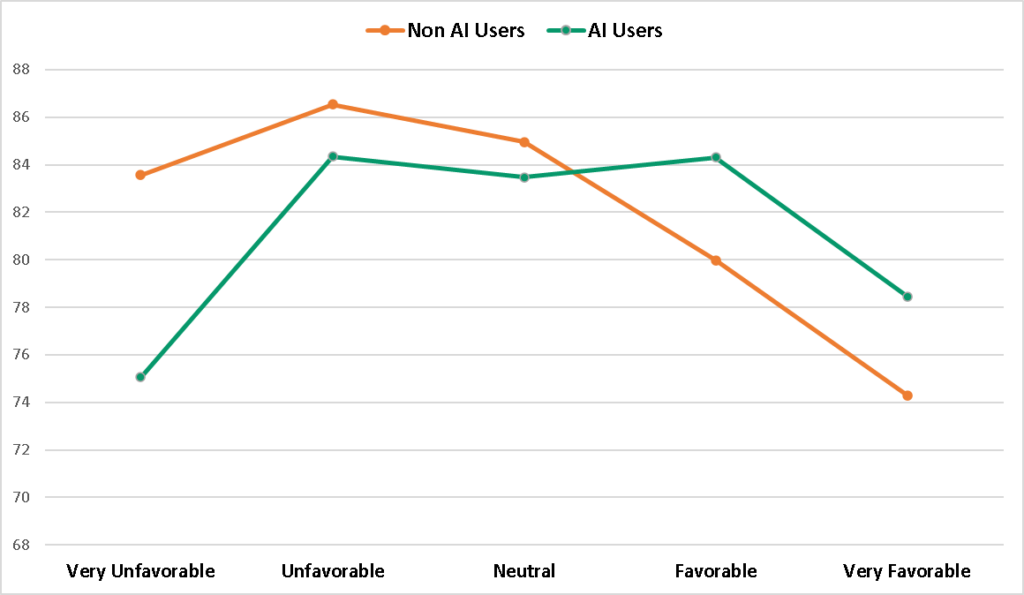
Model 2 – AI users only
This ANCOVA model (R2 = .907) used only AI users for the entire sample. It used the same dependent variable of achievement and covariates of SES, product line, IEP Status, and course load; the only difference was that the major independent variable for this analysis was the AI Use Case variable, which divided AI users into three groups: those who used “AI as a tool,” those who used “AI as a facilitator,” and those who used both.
The ANCOVA results revealed a significant main effect of usage types on student achievement (p =.012, F =7.110), with no other main effects. This means that how students utilized AI alone had an impact on their grades.
Students reporting using AI as only a tool had average grades of 76.5 (std deviation 20.72), while students who reported using AI as both a tool and facilitator had average grades of 83.9 (std deviation 16.23). In addition, a Pearson R correlation was conducted to measure the strength of the relationship between these two variables, resulting in a small but significant direct correlation (r =.291, p<.05)
There was also a two-way interaction between the AI Use Case variable and subject areas. Those in math, science, foreign language, and “other” courses scored much higher than their counterparts when utilizing AI in both manners, while the inverse was true for ELA and Health & PE courses; notably, there were no AI users in Visual and Performing Arts. This can be seen below in Figure 6.
Figure 6. Average Grades by Subject and AI Use Case
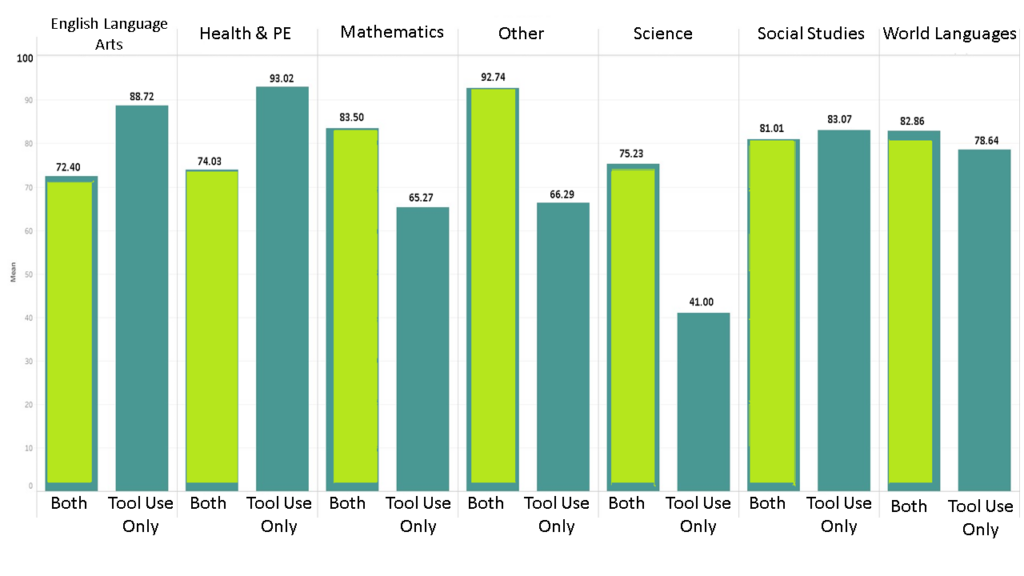
According to these analyses, using AI as both a facilitator and a tool had a significant positive impact on Math and Science scores, or STEM subjects, while this was not the case for all other subjects.
Final Comparisons
After examining grades of AI users and non-AI users, and then within the AI users, a final comparison between the higher achieving group of AI users (those that utilized AI as both a facilitator and a tool and not just as a tool) and non-AI users was conducted.
Results indicated that students who utilized AI as both a tool and a facilitator, on average, outperformed non-AI users. The main effect (p < .05, F= 3.94) of AI use as both a facilitator and tool produced an average grade percentage of 83.9, while non-users reported grades of 82.4. This main effect was present across all subject areas and demographic groups.
Research Question 3: Are there differences in student perceptions on AI?
This research question utilized LMS data and end-of-course survey data in ANCOVA models to address the research question. The independent variables in these models were subject, grade level, and locale, while covariates in the model were a student’s IEP status, SES status, course load, current grade, and product line. The covariates were chosen due to the amount of variance they explained in model iterations, and thus were controlled for accordingly, as the research was focused on variance explained by the other variables.
The dependent variable in this research question was a single item on the end-of-course student survey that asked about students’ perceptions regarding their favorability of AI tools on a 5-point Likert scale. A distribution of the dependent variable is shown below in Figure 7.
Figure 7. AI Perceptions Distribution
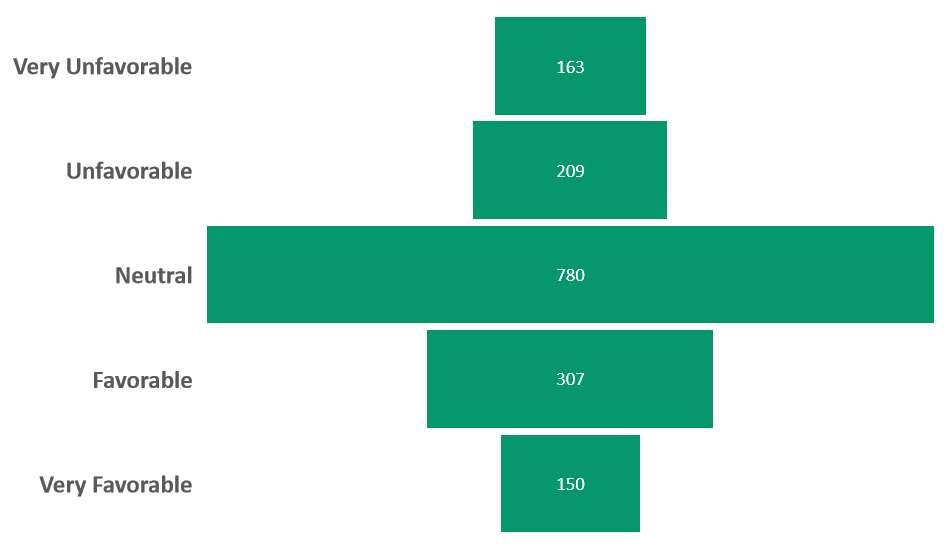
The large majority of students were neutral in their perceptions around AI, with a slight skew to the positive side.
Model 1 – General Model
This ANCOVA model incorporated the aforementioned covariates, and five independent variables: grade level, subject area, location, SES status, and AI usage (R2 =.797).
The variables of AI Usage (p <.001, F =38.92) and the Subject Area (p <.05, F=1.61) had main effects with the dependent variable of AI perception.
Students who utilized AI more had much higher perceptions around AI tools, with those utilizing AI tools reporting an average of around 4, or slightly favorable, and non-users reporting, on average, 2.8 or slightly below the neutral response. This is a large and significant difference.
Additionally, Pearson R values indicate a significant, but moderate direct relationship between the variables (p <.001, r =.333).
Students in English Language Arts, Visual and Performing Arts, and Health and Physical Education Courses rated AI tools lower than students in Math, Science, Language, Social Studies, and other courses. These differences can be seen below in Table 4.
Table 4. Average AI Perceptions by Subject
Subject | Mean | Std. Error |
---|---|---|
English Language Arts | 2.925 | .144 |
Health & Physical Education | 2.665 | .212 |
Mathematics | 3.253 | .124 |
Other | 3.244 | .106 |
Science | 3.458 | .105 |
Social Studies | 3.269 | .097 |
Visual & Performing Arts | 2.903 | .215 |
World Languages | 3.143 | .084 |
These two variables also had a two way interaction ( p =.001, F =3.67), shows that there were differences in users and non users across multiple subjects. These differences can be seen below in Table 5, with significant differences indicated by a (*). Across almost every subject, AI users reported significantly (p <.05) more favorable opinions on AI than non-users.
Table 5 – Average AI Perceptions by Subject and AI Usage
Subject | AI Usage | Average AI Perception | Std. Error |
---|---|---|---|
English Language Arts* | No | 2.570 | .159 |
Yes | 4.192 | .339 | |
Health & Physical Education | No | 2.515 | .219 |
Yes | 3.494 | .63 | |
Mathematics* | No | 2.965 | .139 |
Yes | 3.851 | .247 | |
Other | No | 3.133 | .113 |
Yes | 3.491 | .232 | |
Science* | No | 3.055 | .11. |
Yes | 4.512 | .236 | |
Social Studies* | No | 2.916 | .107 |
Yes | 4.052 | .204 | |
Visual & Performing Arts | No | 2.903 | .215 |
Yes | None | ——— | |
World Languages* | No | 2.809 | .086 |
Yes | 4.161 | .212 |
Model 2 – AI users only
This model utilized the same covariates as Model 1 for RQ3, as well as all of the same independent variables, except for exchanging the AI Usage (yes or no) variable for the AI Use Case variable, categorized users into three bins: those that utilized AI as a tool, as a facilitator, or both.
Results from the model (R2= .781) indicate that there were no main effects on perceptions by any of the independent variables in the model, nor any multiple independent variable interactions with the dependent outcome. There was no significant difference in perceptions of AI with regard to specific use cases amongst AI users.
Research Question 4: What differences exist between student usage of AI?
This research question utilized LMS data and end of course survey data in a general ANCOVA model and an ANCOVA model with AI users only to address the research question. The general ANCOVA model used the single item of AI usage (yes/no response) as the dependent variable, with a subsequent model using the binned AI Use Cases as a single dependent variable.
Findings from research question 1 indicated that only about 8% of students reported utilizing AI.
Model 1 – ANCOVA – General Model
The general model (R2 = .479) utilized AI Usage as the dependent variable, four covariates (IEP, Product Line, Course Load, and Current Grade/Ability), and five independent variables: AI Perception, subject, grade level, locale, and SES.
There was a main effect interaction of opinion on the binary dependent variable of AI Usage ( p< .001, F =11.120). Those students with more favorable opinions utilized AI more, which is shown below in Figure 8. This is consistent with the findings from the previous research question; Pearson R values indicated a significant, but moderate direct relationship between the variables (p <.001, r =.333).
Figure 8 – AI Perception and Utilization
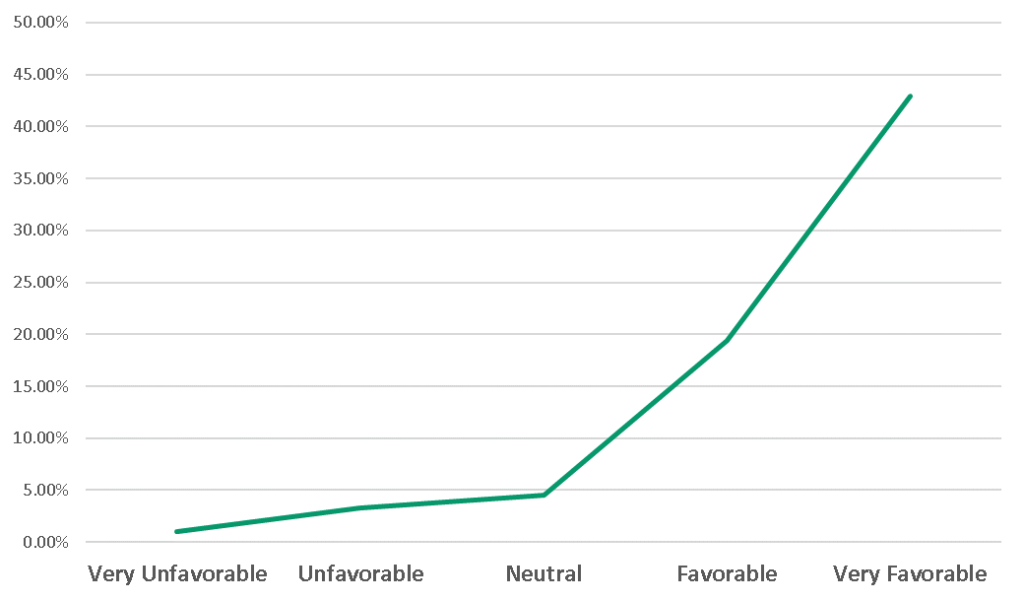
Students that held Very Favorable opinions about AI utilized it almost 50% of the time on average, while students with Very Unfavorable opinions utilized AI only 1% of the time on average; this is a very large difference, fifty times more utilization reported between top and bottom bins.
Model 2 ANCOVA – AI users only
This model (R2 =.642) utilized the binned AI Use Case variable as the dependent, which categorized the original multiple response variables that contained multiple dependents into a single variable with three categories: AI used as a tool, AI used as a facilitator, or both. All other independent variables and covariates from the general model were the same.
This model found no significant differences between groups, nor any multi-independent interactions, meaning that there were no main effects of independent variables on the use cases of which students were utilizing AI tools.
Synthesis
Finding 1 (Usage)
Student usage of AI tools was minimal, slightly less than 10% of students self-reported utilizing AI tools for their online learning courses. Though this seems low, measures were taken to inform all students that indication of their AI use would not penalize them in any way.
The most largely utilized tool by students at the time of this study was ChatGPT.
All students that used AI indicated that they made use of AI as tool, or like a tool, while over 2/3s of AI users stated that they utilized AI in ways that were like a tool and as a facilitator in learning, meaning that no users only utilized AI as a facilitator.
The usage of AI tools alone had little to no impact on the student outcome of student achievement. The ways in which students utilized AI showed differences in achievement based on the types of use cases students reported. Students who utilized AI as both a facilitator and a tool had better achievement outcomes than students who utilized AI as only a tool, though it is not consistent across all subject types, and correlation data indicates that there is a small, but significant relationship (r =.291, p<.05) between usage types and grades.
Students in STEM subjects (including Computer Science from the “other” category of courses) that utilized AI in both manners outperformed their counterparts that used it only as a tool— this was not the case in all other subjects. In addition, those students who utilized AI as both a facilitator and a tool outperformed the non-AI users by a margin of 2.5 points across all subjects, on average.
Finding 2 (Perceptions)
Students, on average, tend to have more neutral views regarding AI as opposed to extreme views, and those with the extreme views tend to have worse student outcomes. There may be a nonlinear relationship between the variables of achievement and AI perceptions, but this study only revealed the slight, but significant, presence of such a relationship.
The relationship between usage and perceptions around AI is significant, those students who use AI tools tend to have better perceptions of it, and vice versa, which was supported by hypothesis testing and correlational data. However, amongst AI users, how students utilized AI made no difference in how they perceived it.
Key Points
- Using artificial intelligence alone does not have a strong relationship with student achievement.
- Simply using AI alone doesn’t make students perform better or worse
- Many students aren’t utilizing the AI for school at all, or at least reporting usage.
- The way in which students use AI tools matters
- Students who use AI in multifaceted facilitative use cases in addition to any lower-level use cases have significantly better grades than students who strictly utilize AI as a tool for lower-level use cases on average, but subjects vary.
- This is strongly present in STEM subjects, but not as clear for others.
- Students in English courses with AI “as a tool” only scored higher than those that used it for both, which may mean that AI usage “as a tool” is more effective in that area for ELA assignments, such as writing.
- Students who use AI as a tool and a facilitator outperform non-AI user counterparts on average.
- Students who use AI in multifaceted facilitative use cases in addition to any lower-level use cases have significantly better grades than students who strictly utilize AI as a tool for lower-level use cases on average, but subjects vary.
- AI perceptions differ across and within subject areas.
- STEM subjects, social studies, and foreign languages had higher perceptions than other subjects.
- Students who have less extreme perceptions of AI tend to have better student outcomes. There is a very small but significant quadratic relationship between the variables that should be investigated further.
- Students who utilize AI more have better perceptions regarding its usefulness.
- This was mostly consistent across subject areas.
- SES and locales as non-factors
- While a student’s SES was shown to have a main effect on student achievement, its variance was appropriately accounted for by inclusion as a covariate in subsequent models with achievement as a dependent, as this is a common factor with achievement; research has found repeatedly that low SES students usually achieve lower than their higher SES counterparts. Other models that did not use achievement as the dependent included SES as an independent variable in order to see if its effect was still present, and results indicate that it was not.
- A student’s locale was also not a significant factor in any of the models, nor were any models adjusted to co-vary on its contribution to variance in the dependents.
Conclusions
This section will discuss the results of the study alongside existing literature, as well as provide recommendations for policymakers and future research on the topic of student AI usage.
Multiple tables at the end of this section will organize recommendations with resources for practitioners and policymakers, but a comprehensive list of resources curated by Michigan Virtual can be found here.
Implications for Practice
This section discusses how the findings of this study fit into the larger context of education as a whole by comparing and contrasting results to the existing literature and examining where it fits in current practice.
Encourage multifaceted and facilitative use of AI tools: Educators should promote the use of AI technologies not just as a tool but also as a facilitator in the learning process. Students who utilized AI as both a facilitator and a tool had better achievement outcomes compared to those who used it solely as a tool. This type of approach is supported by Luckin et al. (2016), because it focuses on utilizing AI as an assistant to promote critical thinking and creative problem solving rather than shortcutting students to answers. Additionally, the distribution of use cases found in this study are consistent with findings from other large scale studies (Walton Family Foundation, 2024), compounding evidence that these types of use cases are accurate.
Therefore, measures should be taken to provide guidance on how to effectively integrate AI as a facilitator of learning, at the very least including the categories in this study: to explain complicated concepts or principles in simpler terms, to create study guides or sample test questions, and using AI as tutor / teacher.
Address extreme perceptions of AI: The study found that students with extreme views (either positive or negative) regarding AI tend to have worse student outcomes. This is consistent with findings that show that perceptions of students are varied (Martinez et. al, 2023; Diliberti, Schwartz, Doan, Shapiro, Rainey, & Lake , 2024; Trisoni et al., 2023; Walton Family Foundation, 2024). However while perceptions were varied, they tended to be normally distributed, and mostly neutral, which is different from the positive leaning perceptions among students found by the Walton Family Foundation (2024) study. According to this study, while opinions may be varied, education should aim to cultivate a balanced and informed understanding of AI among students to promote better outcomes.
Equity in AI Usage: This study did not indicate that there was any disparity amongst AI usage regarding students of lower socioeconomic status or locale; there were no interactions between the variable of SES and other independent variables related to AI usage or perceptions. This is consistent with findings regarding locale in the Walton Family Foundation study (2024). This is evidence that students in online learning environments, regardless of socioeconomic status, likely have access to some form of AI tools, whether or not they choose to utilize them.
Subject specific Utilization of AI and Opinions of AI : As with adults (McGehee 2023), this study indicates that students who use AI more tend to have better opinions of it, and vice versa. Perceptions across subject levels with students vary, with students in STEM subjects tending to have higher perceptions of AI, and higher scoring students that utilized AI. This is consistent with the idea presented by Zawacki-Richter et al. (2022) that AI may be useful in promoting development of “hard” skills like critical thinking and problems solving that are central to STEM.
Age, grade level, and environment: This study consisted of a sample of students in asynchronous online learning environments, primarily in high school grades. This means that the results cannot be generalized to populations that are not similar; students in differing environments and grade levels may report different results.
However, at the time of this study, this sample size is one of the largest recorded that deals with student AI usage, and many of the results are similar to other studies, with the exception that this sample has reported lower student AI usage overall compared to the Walton Family Foundation study (2024).
Future Research: More research is needed in both of the focus areas of this study. While utilizing a large dataset, the data is very surface level and does not help us understand a lot of the contextual questions that the findings raised. The sample is also focused on a population of secondary school students in asynchronous, online learning environments.
Future studies would benefit from better instrumentation and data collection protocols to expand on the findings of this study to understand how students utilize AI, what they think about AI, and what relationships those things have with outcomes that concern stakeholders. Below are specific examples:
- Conduct further research on usage habits, specifically amongst different types of learning environments.
- Investigate specific use cases of AI in specific subjects with controlled variables and environments.
- Qualitative research regarding student AI perceptions and use cases.
- Utilization of AI and high stakes testing.
- Effectiveness of AI integration into coursework from both teacher and student perspectives.
- Prevalence of cheating using AI.
- Investigate changes in usage percentages and usage percentages over time.
- AI usage is largely in its infancy at the time of this study, and these findings are likely to change as it becomes more prevalent.
Recommendations
For Policymakers:
- Develop guidelines and provide training for effective AI integration: Since the way students use AI tools matters, policymakers should develop guidelines and provide training for teachers on how to integrate AI tools into the curriculum effectively. These guidelines should emphasize the importance of using AI in multifaceted and facilitative ways, rather than just as a tool for lower-level tasks.
- Encourage subject-specific approaches to AI integration: The findings suggest that the impact of AI usage may differ across subject areas, with STEM subjects benefiting from facilitative AI usage, while ELA and PE courses might require different approaches. Policymakers should encourage subject-specific strategies for AI integration and provide resources accordingly.
- Promote AI literacy and balanced perceptions: Students with less extreme perceptions of AI tend to have better outcomes. Policymakers should develop initiatives to promote AI literacy and foster balanced perceptions of AI among students. This could involve incorporating AI education into curricula or organizing awareness campaigns.
- Support research on AI usage and perceptions: Further research is needed to understand the quadratic relationship between AI perceptions and student outcomes. Policymakers should allocate funding and resources for continued research in this area to inform evidence-based practices.
- Ensure equitable access to AI tools: While SES and locale were not significant factors affecting AI usage or perceptions, policymakers should still ensure that all students, regardless of their socioeconomic status or location, have equitable access to AI tools and resources. This study is a single source of evidence to support the idea that AI access may not be limited to the wealthy, but it is still a valid concern, as socioeconomic status has historically been a risk factor.
Policy Recommendation | Resources |
---|---|
Develop guidelines and provide training for effective AI tool integration | Michigan Virtual’s AI Integration Framework Demystifying AI by All4Ed Michigan Virtual’s K-12 AI Guidelines for Districts YouTube Video: District Planning Michigan Virtual Workshops |
Encourage subject-specific approaches to AI integration | Michigan Virtual Workshops |
Promote AI literacy and balanced perceptions | Michigan Virtual’s AI Integration Framework Demystifying AI by All4Ed |
Support research on AI usage and perceptions | Partner with local universities and research organizations, like Michigan Virtual! |
Ensure equitable access to AI tools | Michigan Virtual AI Planning Guide |
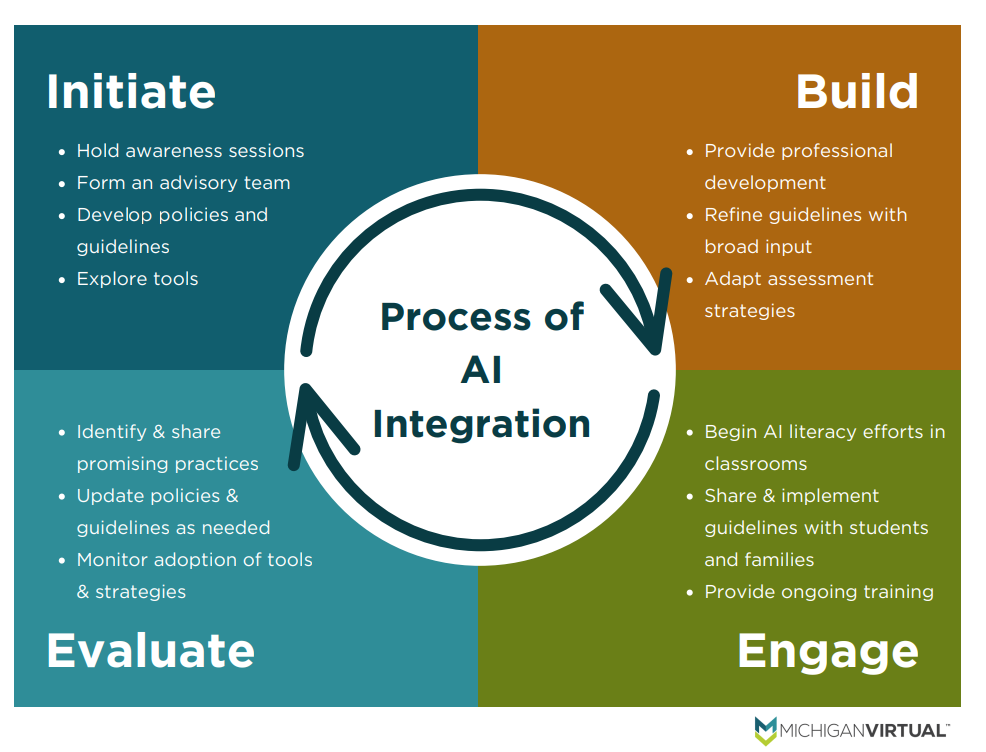
For Teachers:
- Integrate AI tools in multifaceted and facilitative ways: Teachers should strive to incorporate AI tools not just as tools for lower-level tasks but also as facilitators for learning. This could involve using AI for tasks like personalized feedback, interactive simulations, or collaborative projects. Guidance for how to avoid student reliance on AI and encourage facilitative use can be found here.
- Adapt AI integration strategies based on subject area: Teachers should be aware that the impact of AI usage may differ across subject areas. They should adapt their strategies for AI integration based on the specific needs and requirements of their subject area.
- ELA teachers should especially adapt assignments that encourage higher-order skills so that simple AI “tool-use” will not result in plagiarism, dishonesty, or a lack of quality learning (or lower achievement).
- STEM teachers should promote the utilization of AI as a facilitator and differentiator to support their students’ learning.
- Address student perceptions and promote balanced views: Teachers should actively address student perceptions and misconceptions about AI. They should aim to foster balanced and informed views by providing accurate information, addressing concerns, and highlighting both the potential benefits and limitations of AI.
- Collaborate with colleagues and seek professional development: Teachers should collaborate with colleagues, particularly those teaching different subject areas, to share best practices and learn from each other’s experiences with AI integration. They should also seek professional development opportunities to enhance their skills in effectively using AI tools in the classroom.
- Provide guidance and support for AI tool usage: Since many students are not utilizing AI tools or reporting usage, teachers should provide guidance and support to encourage appropriate and effective AI tool usage among students.
Teacher Recommendation | Resources |
---|---|
Integrate AI tools in multifaceted and facilitative ways | Michigan Virtual Student AI Use Cases YouTube Series: Practical AI for Instructors and Students YouTube Video: Using AI for Instructional Design Teaching with AI (Open AI) |
Adapt AI integration strategies based on subject area | Michigan Virtual Student AI Use Cases AI in the Classroom |
Address student perceptions and promote balanced views | Next Level Labs Report: Demystify AI NSF AI Literacy Article |
Collaborate with colleagues and seek professional development | Michigan Virtual Student AI Use Cases Youtube Series: Practical AI for Instructors and Students YouTube Video: Using AI for Instructional Design Teaching with AI (Open AI) |
Provide guidance and support for AI tool usage | YouTube Series: Practical AI for Instructors and Students Next Level Labs Report Youtube Video: ChatGPT Prompt Writing AI 101 for Teachers Michigan Virtual Courses |
References
Beck, J., Stern, M., & Haugsjaa, E. (1996). Applications of AI in Education. XRDS: Crossroads, The ACM Magazine for Students, 3(1), 11-15.
Bender, E. M., Gebru, T., McMillan-Major, A., & Shmitchell, S. (2021). On the dangers of stochastic parrots: Can language models be too big? In Proceedings of the 2021 ACM Conference on Fairness, Accountability, and Transparency (pp. 610-623).
Chen, Y., Jensen, S., Albert, L. J., Gupta, S., & Lee, T. (2023). Artificial intelligence (AI) student assistants in the classroom: Designing chatbots to support student success. Information Systems Frontiers, 25(1), 161-182.
Diliberti, M. K., Schwartz, H. L., Doan, S., Shapiro, A., Rainey, L. R., & Lake, R. J. (2024). Using artificial intelligence tools in K–12 classrooms. RAND Corporation. https://www.rand.org/pubs/research_reports/RRA956-21.html
Driessen, G., & Gallant, T. B. (2022). Academic integrity in an age of AI-generated content. Communications of the ACM, 65(7), 44-49.
Freidhoff, J. R., DeBruler, K., Cuccolo, K., & Green, C. (2024). Michigan’s k-12 virtual learning effectiveness report 2022-23. Michigan Virtual. https://michiganvirtual.org/research/publications/michigans-k-12-virtual-learning-effectiveness-report-2022-23/
Kulik, J. A., & Fletcher, J. D. (2016). Effectiveness of intelligent tutoring systems: a meta-analytic review. Review of Educational Research, 86(1), 42-78.
Kumar, V. R., & Raman, R. (2022). Student Perceptions on Artificial Intelligence (AI) in higher education. In 2022 IEEE Integrated STEM Education Conference (ISEC) (pp. 450-454). IEEE.
Luckin, R., Holmes, W., Griffiths, M., & Forcier, L. B. (2016). Intelligence Unleashed: An argument for AI in Education. https://www.pearson.com/content/dam/corporate/global/pearson-dot-com/files/innovation/Intelligence-Unleashed-Publication.pdf
Martínez, I. G., Batanero, J. M. F., Cerero, J. F., & León, S. P. (2023). Analysing the impact of artificial intelligence and computational sciences on student performance: Systematic review and meta-analysis. NAER: Journal of New Approaches in Educational Research, 12(1), 171-197.
Mehrabi, N., Morstatter, F., Saxena, N., Lerman, K., & Galstyan, A. (2021). A survey on bias and fairness in machine learning. ACM Computing Surveys (CSUR), 54(6), 1-35.
Selwyn, N. (2019). Should robots replace teachers? AI and the future of education. John Wiley & Sons.
Trisoni, R., Ardiani, I., Herawati, S., Mudinillah, A., Maimori, R., Khairat, A., … & Nazliati, N. (2023, November). The Effect of Artificial Intelligence in Improving Student Achievement in High Schools. In International Conference on Social Science and Education (ICoeSSE 2023) (pp. 546-557). Atlantis Press.
Walton Family Foundation. (2024). AI Chatbots in Schools. https://www.waltonfamilyfoundation.org/learning/the-value-of-ai-in-todays-classrooms
Winkler, R., & Söllner, M. (2018). Unleashing the Potential of Chatbots in Education: A State-Of-The-Art Analysis. In Academy of Management Annual Meeting (AOM).
Zawacki-Richter, O., Marín, V. I., Bond, M., & Gouverneur, F. (2019). Systematic review of research on artificial intelligence applications in higher education – where are the educators?. International Journal of Educational Technology in Higher Education, 16(1), 1-27.
Zheng, L., Niu, J., Zhong, L., & Gyasi, J. F. (2023). The effectiveness of artificial intelligence on learning achievement and learning perception: A meta-analysis. Interactive Learning Environments, 31(9), 5650-5664.